Home>Home Security and Surveillance>How To Find New Results For Intrusion Detection System Research
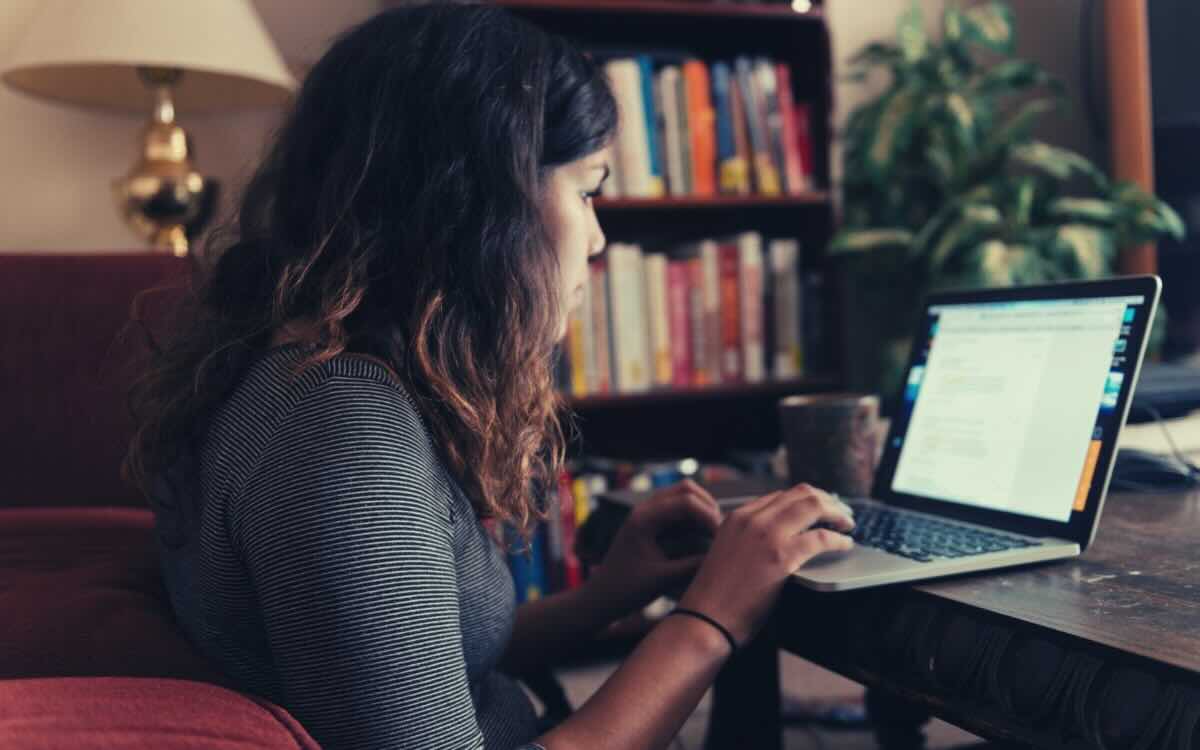
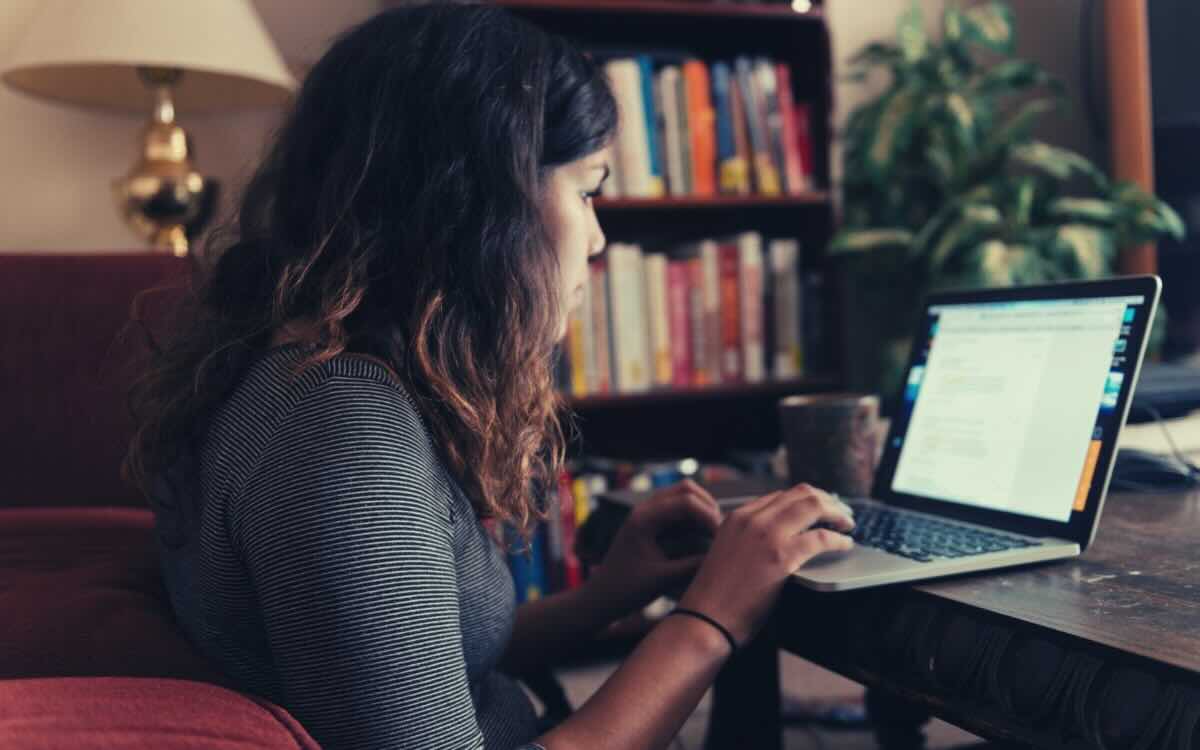
Home Security and Surveillance
How To Find New Results For Intrusion Detection System Research
Modified: October 21, 2024
Looking for new results for intrusion detection system research? Discover how home security and surveillance can enhance your research in this comprehensive guide.
(Many of the links in this article redirect to a specific reviewed product. Your purchase of these products through affiliate links helps to generate commission for Storables.com, at no extra cost. Learn more)
Introduction
Welcome to the world of home security and surveillance! In today’s fast-paced and connected world, ensuring the safety of our homes and loved ones has become more important than ever. With advancements in technology, home security systems have evolved to provide sophisticated solutions that not only detect and prevent intrusions but also offer peace of mind to homeowners.
Intrusion detection systems (IDS) are a crucial component of any home security setup. These systems are designed to detect and alert homeowners about any unauthorized access or suspicious activities within their premises. From traditional motion sensors to smart cameras equipped with artificial intelligence, the options are expansive and constantly evolving.
In this article, we will delve into the world of IDS research and explore strategies for finding new results in this field. By constantly seeking innovative approaches and staying up-to-date with the latest advancements, researchers can contribute to the development of more robust and effective intrusion detection systems.
Whether you are a student exploring this field, an industry professional looking to enhance security measures, or simply a curious enthusiast, this article will provide valuable insights into the fascinating world of IDS research.
So, let’s dive in and uncover the possibilities for finding new results in intrusion detection system research!
Key Takeaways:
- Stay Ahead of Cyber Threats
Researchers in home security and surveillance must constantly seek new results to develop advanced intrusion detection systems. By exploring innovative approaches and collaborating with industry experts, they can stay ahead of emerging cyber threats and protect homes and loved ones. - Embrace Emerging Technologies
Exploring cutting-edge technologies such as artificial intelligence and machine learning is crucial for enhancing intrusion detection systems. Researchers can leverage these advancements to improve detection accuracy, scalability, and response strategies, contributing to a safer digital environment.
Read more: How To Test Intrusion Detection System
Background of Intrusion Detection System (IDS) Research
Intrusion Detection Systems (IDS) have been a subject of extensive research and development for several decades. Their primary objective is to detect and prevent unauthorized access, attacks, or malicious activities within a network or a system. IDS research has played a pivotal role in advancing home security and surveillance technology, making it more intelligent and effective.
The origins of IDS research can be traced back to the early days of computer networks. As the internet grew in popularity, so did the threats associated with it. Traditional security measures, such as firewalls and antivirus software, proved insufficient in detecting sophisticated attacks that could compromise the confidentiality, integrity, and availability of data.
IDS research emerged as a response to the evolving threat landscape, aiming to develop advanced techniques for identifying and responding to unauthorized activities. Over the years, IDS research has focused on several key areas:
- Signature-based IDS: Signature-based IDS systems use predefined patterns or signatures to detect known attacks. These signatures are based on the characteristics of previously identified threats and are stored in a database. When incoming network traffic matches a signature, an alert is triggered. While signature-based IDS systems are effective in detecting known attacks, they are limited in their ability to identify new or unknown threats.
- Anomaly-based IDS: Anomaly-based IDS systems analyze network traffic or system behavior to establish a baseline of normal activities. Any deviation from this normal pattern is flagged as a potential intrusion. Anomaly-based IDS research focuses on developing algorithms and machine learning techniques to accurately identify anomalous behavior while minimizing false positives.
- Hybrid IDS: Hybrid IDS systems combine the strengths of both signature-based and anomaly-based approaches. They leverage signatures to detect known attacks and employ anomaly detection techniques to identify new or unknown threats. Hybrid IDS research aims to find the optimal balance between accuracy and efficiency.
Though significant progress has been made in IDS research, challenges remain. Cybercriminals are constantly evolving their attack techniques to bypass traditional IDS systems. Moreover, the increasing complexity and scale of modern networks require IDS systems that can handle large volumes of data in real-time.
As a result, there is a constant need for new ideas, approaches, and innovations to improve the effectiveness, efficiency, and adaptability of intrusion detection systems. Researchers play a critical role in pushing the boundaries of IDS technology, seeking new results that can address the evolving threat landscape and protect homes and businesses from ever-changing security risks.
In the following sections, we will explore strategies that researchers can employ to find new results in IDS research, paving the way for more advanced and robust intrusion detection systems.
Traditional Approaches in IDS Research
Traditional approaches in intrusion detection system (IDS) research have laid the foundation for the development of effective security measures. These approaches, though fundamental, have played a vital role in enhancing the security posture of networks and systems. Let’s explore some of the traditional approaches in IDS research:
- Rule-Based Systems: Rule-based IDS systems use a set of predefined rules to identify malicious activities. These rules are created based on known attack signatures or patterns. When network traffic matches any of the rules, an alert is triggered. Rule-based systems are widely used and have proven effective in detecting well-known attacks. However, they struggle to detect new or unknown threats that do not match the existing set of rules.
- Statistical Analysis: Statistical analysis approaches involve analyzing network traffic or system logs to establish baseline behavior. Deviations from the baseline are flagged as potential anomalies or intrusions. Statistical methods, such as anomaly detection algorithms and time-series analysis, are employed to identify abnormal patterns. While statistical analysis can help detect previously unseen attacks, it can also lead to a high number of false positives, requiring further refinement and optimization.
- Machine Learning: Machine learning techniques have gained significant traction in IDS research. These approaches involve training models using labeled datasets comprising normal and malicious activities. The trained models can then classify new data as either normal or malicious. Machine learning algorithms, such as decision trees, support vector machines, and neural networks, have shown promise in identifying complex attack patterns and adapting to new threats. However, machine learning approaches require large amounts of diverse and high-quality training data to build accurate models, and they can be vulnerable to adversarial attacks.
- Data Mining: Data mining approaches involve extracting meaningful insights and patterns from large volumes of data. IDS research leverages data mining techniques to identify associations, clusters, and anomalies in network traffic or system logs. By exploring hidden patterns, data mining can help uncover indicators of compromise and improve the accuracy of intrusion detection. However, data mining approaches can be computationally intensive and require skilled analysts to interpret the results effectively.
While these traditional approaches have made significant contributions to IDS research, they have their limitations. Traditional IDS systems often struggle to keep up with the rapidly evolving threat landscape, where attackers constantly devise new techniques to evade detection. Additionally, the volume and complexity of data in modern networks can overwhelm traditional approaches, leading to false positives or missed detections.
To overcome these limitations and find new results in IDS research, researchers are exploring innovative strategies and integrating emerging technologies into existing approaches. In the next sections, we will explore these strategies in detail and uncover new avenues for advancing intrusion detection systems.
Limitations of Existing IDS Research
While intrusion detection systems (IDS) have come a long way in terms of improving the security of networks and systems, there are still several limitations that researchers need to address. These limitations highlight the need for constant innovation and the pursuit of new results in IDS research. Let’s explore some of the key limitations of existing IDS research:
- False Positives and False Negatives: IDS systems often suffer from the trade-off between false positives and false negatives. False positives occur when benign activities are flagged as potential intrusions, leading to unnecessary alarms and wasted resources. False negatives, on the other hand, occur when actual intrusions go undetected. Striking a balance between minimizing false positives and false negatives is a constant challenge that researchers face.
- Limited Detection Capabilities: Existing IDS systems primarily focus on detecting known attacks or predefined patterns. While these signature-based approaches are effective in identifying known threats, they struggle to detect new or unknown attacks. Zero-day exploits and sophisticated evasion techniques can easily bypass signature-based IDS systems, making them less reliable in an ever-changing threat landscape.
- Scalability: With the exponential growth of data in modern networks, IDS systems face significant challenges in terms of scalability. Traditional IDS research often struggles to handle large volumes of network traffic in real-time, leading to performance bottlenecks and delays in detection. As networks expand in size and complexity, IDS solutions need to scale effectively to accommodate the increased traffic and maintain efficient detection capabilities.
- Difficulty in Handling Encrypted Traffic: Encrypted traffic poses a significant challenge for IDS systems. While encryption is crucial for securing data transmission, it also hampers the ability of IDS systems to inspect network packets for malicious activities. Intrusions hidden within encrypted traffic can go undetected, making it necessary for researchers to develop innovative solutions that can effectively analyze encrypted traffic without compromising privacy.
- Adaptability to Evolving Threats: Attackers are constantly evolving their techniques to bypass IDS systems. Researchers need to stay one step ahead and develop IDS solutions that can adapt to new and emerging threats. This requires continuous monitoring of the threat landscape, collaboration with experts, and the incorporation of innovative approaches such as machine learning, artificial intelligence, and behavioral analysis into existing IDS systems.
- Complexity of Analysis: IDS research often involves complex analysis of network traffic, system logs, and other data sources. Interpreting and analyzing this data can be challenging, requiring skilled analysts and advanced tools. Researchers need to develop user-friendly interfaces and visualization techniques that can simplify the analysis process and enable timely and accurate detections.
Addressing these limitations is critical for advancing IDS research and improving the effectiveness of intrusion detection systems. By seeking new results and embracing innovative approaches, researchers can pave the way for more robust, intelligent, and adaptive IDS solutions that can effectively detect and respond to emerging threats.
In the next sections, we will explore strategies for finding new results in IDS research and overcoming these limitations to enhance the security of homes and businesses.
Importance of Seeking New Results for IDS Research
Seeking new results in intrusion detection system (IDS) research is of paramount importance for several reasons. With the ever-evolving threat landscape and the increasing complexity of network environments, researchers must constantly push the boundaries of IDS technology to enhance the security of homes and businesses. Let’s explore the importance of seeking new results in IDS research:
- Staying Ahead of Emerging Threats: Attackers are constantly devising new techniques and strategies to bypass traditional security measures. By seeking new results, researchers can develop innovative approaches that can effectively detect and mitigate emerging threats. This proactive mindset allows IDS systems to stay one step ahead of attackers, ensuring the security of networks and systems.
- Enhancing Detection Accuracy: IDS research focuses on improving detection accuracy by minimizing false positives and false negatives. By exploring new methods, researchers can develop more refined algorithms, machine learning models, and data-driven approaches to accurately identify and classify malicious activities. Improved detection accuracy leads to more reliable and efficient IDS systems.
- Adapting to Evolving Technologies: The rapid advancement of technology introduces new challenges and opportunities in the field of IDS research. Researchers must explore new solutions that can handle emerging technologies such as cloud computing, Internet of Things (IoT), and virtualization. Seeking new results allows researchers to develop IDS systems that are compatible with modern network environments and can effectively detect intrusions across diverse platforms.
- Addressing Limitations of Existing IDS Approaches: Existing IDS approaches often have limitations in terms of scalability, handling encrypted traffic, and detecting unknown threats. By seeking new results, researchers can address these limitations and find innovative solutions. For example, incorporating artificial intelligence and machine learning techniques can improve scalability and enhance the ability to detect unknown attacks effectively.
- Improving Response and Mitigation: Intrusion detection is not only about identifying malicious activities but also about responding quickly and effectively to mitigate the impact of intrusions. Seeking new results allows researchers to develop advanced response mechanisms, automated incident handling techniques, and intelligent mitigation strategies. This ensures that IDS systems not only detect intrusions but also take prompt action to minimize damage and protect critical assets.
- Contributing to the Security Community: IDS research plays a crucial role in advancing the overall security community. By seeking new results, researchers contribute to the collective knowledge, sharing insights, and findings that can benefit other researchers, industry professionals, and end-users. This collaborative effort fosters a stronger security ecosystem, driving innovation and improving the security posture of homes, businesses, and organizations.
Overall, seeking new results in IDS research is vital for adapting to the evolving threat landscape, improving detection accuracy, addressing limitations of existing approaches, and enhancing the security of networks and systems. By constantly exploring new ideas, technologies, and methodologies, researchers can make significant contributions to the field of intrusion detection and help create a safer digital environment for all.
In the next sections, we will dive into specific strategies that researchers can employ to find new results in IDS research.
Read more: How To Create Intrusion Detection System
Strategies for Finding New Results for IDS Research
To find new results and push the boundaries of intrusion detection system (IDS) research, researchers can employ various strategies that foster innovation, collaboration, and exploration. These strategies enable researchers to uncover novel approaches, techniques, and insights that can advance the field of IDS. Let’s explore some effective strategies for finding new results in IDS research:
- Literature Review: Conducting a thorough literature review is essential in staying up-to-date with the latest advancements in IDS research. By reviewing academic papers, industry reports, and conference proceedings, researchers can identify gaps in existing knowledge and uncover new research directions. This strategy allows researchers to build upon previous work and identify emerging trends in intrusion detection.
- Collaboration with Industry Experts: Collaborating with industry experts and professionals is a valuable strategy for finding new results in IDS research. By engaging with practitioners and security professionals, researchers can gain insights into real-world challenges, industry best practices, and emerging threats. Collaborative efforts between researchers and industry experts foster knowledge exchange and ensure that IDS research remains relevant and effective in practical scenarios.
- Experimentation with Novel Techniques: Researchers can explore new techniques and methodologies by conducting experiments in controlled environments. This strategy involves designing and implementing novel intrusion detection algorithms, machine learning models, or data analysis techniques. Through experimentation, researchers can evaluate the effectiveness and efficiency of these new approaches and gain valuable insights into their potential applications.
- Analysis of Real-World Intrusion Data: Analyzing real-world intrusion data is a rich source of information for finding new results in IDS research. Researchers can collaborate with organizations or security agencies to access and analyze anonymized network traffic, system logs, or attack traces. This analysis can reveal patterns, trends, and characteristics of real intrusions, helping researchers to develop more accurate and targeted detection strategies.
- Exploration of Emerging Technologies: Keeping abreast of emerging technologies is crucial in IDS research. Researchers can explore the potential of technologies such as artificial intelligence, machine learning, behavior-based analysis, and threat intelligence platforms. By integrating these emerging technologies into IDS systems, researchers can enhance the detection capabilities and improve response mechanisms.
By employing these strategies, researchers can actively contribute to the advancement of IDS research and find new results that enhance the effectiveness, efficiency, and adaptability of intrusion detection systems. It is important to continuously explore new ideas, methodologies, and technologies to stay at the forefront of IDS research and address the evolving challenges of the digital landscape.
In the next section, we will conclude our exploration of IDS research and highlight the importance of constantly seeking new results in this dynamic field.
To find new results for intrusion detection system research, explore emerging technologies like machine learning and artificial intelligence for more advanced and accurate detection methods.
Literature Review
A literature review is an essential strategy for finding new results in IDS (Intrusion Detection System) research. It involves a comprehensive examination and analysis of existing academic papers, conference proceedings, industry reports, and other relevant publications. By conducting a literature review, researchers can identify gaps in current knowledge, gain insights into established methodologies, and uncover emerging trends and directions in IDS research.
Here are some key steps to effectively conduct a literature review for IDS research:
- Identification of Key Research Areas: Start by identifying the key research areas within the field of IDS. These may include topics such as signature-based detection, anomaly detection, machine learning techniques, behavior-based analysis, or network traffic analysis. Understanding the different aspects and sub-areas of IDS research will help guide your literature search.
- Selection of Relevant Sources: Identify and select relevant sources of information for your review. This may include academic journals, conference proceedings, research papers, and industry reports. Consider both recent and influential publications to ensure you have a comprehensive understanding of the current state of IDS research.
- Keyword Search and Retrieval: Use appropriate keywords and search techniques to retrieve relevant literature. These keywords can include terms such as “intrusion detection system,” “anomaly detection,” “machine learning IDS,” and other relevant phrases. Utilize research databases, online libraries, and search engines to retrieve academic papers and other publications related to your research area.
- Screening and Evaluation: Screen and evaluate the retrieved literature to determine its relevance and quality. Read abstracts and summaries to assess if the papers align with your research objectives. Focus on high-quality publications and influential works that have been cited by other researchers in the field. This will ensure you are reviewing the most impactful research.
- Analysis and Synthesis: Analyze the literature to identify common themes, trends, and findings. Look for gaps or areas where existing research has not adequately addressed specific issues or challenges. Compare and contrast different approaches, methodologies, and evaluation techniques used in the literature. This analysis will help you identify areas where new results can be sought and contribute to the existing body of knowledge.
- Identifying Research Questions: As you review the literature, identify research questions or areas where new results can be pursued. These research questions can be specific challenges within a certain aspect of IDS, unexplored avenues in existing approaches, or emerging trends that require further investigation. Clearly defining these research questions will guide your future research and contribute to finding new results.
- Synthesizing and Documenting Findings: Finally, synthesize and document your findings from the literature review. Summarize the key insights, trends, and research gaps you have identified. These synthesized findings will serve as a foundation for your own research and will help shape your research objectives, methodologies, and evaluation techniques.
By conducting a thorough literature review, researchers can build upon existing knowledge, identify research gaps, and find new directions for their IDS research. Literature reviews provide a valuable starting point for any research project and ensure that researchers have a comprehensive understanding of the field before embarking on their own investigations.
In the next section, we will explore another strategy for finding new results in IDS research: collaboration with industry experts.
Collaboration with Industry Experts
Collaboration with industry experts is a valuable strategy for finding new results in IDS (Intrusion Detection System) research. By engaging with professionals and practitioners working in the field of cybersecurity and home security, researchers can gain valuable insights, practical knowledge, and real-world perspectives that can enhance their research and contribute to the development of more effective intrusion detection systems.
Here are some key benefits and strategies for collaborating with industry experts in IDS research:
- Real-World Challenges: Industry experts possess firsthand knowledge of the challenges and issues faced in real-world security scenarios. By collaborating with these experts, researchers can gain insights into specific problems and complexities encountered in practical settings. This understanding of real-world challenges helps researchers address the practical applicability and effectiveness of their IDS research.
- Domain Expertise: Professionals working in the industry bring domain-specific expertise and experience that complements the academic research perspective. Collaboration with industry experts allows researchers to leverage this expertise and gain a deeper understanding of the industry’s requirements, constraints, and best practices. This knowledge aids in developing IDS systems that are more aligned with industry needs.
- Data and Resources: Industry experts often have access to large volumes of real-world data, including network traffic logs, attack traces, and intrusion patterns. Collaborating with these experts provides researchers with access to valuable datasets that can be used for training, evaluating, and validating IDS models and techniques. Industry experts can also provide resources, infrastructure, and tools that researchers may not have access to.
- Validation and Feedback: Industry experts can serve as valuable validators of research findings and can provide feedback on the practical viability and deployability of new IDS approaches. Their insights and evaluations can help researchers refine their ideas, validate their assumptions, and ensure that the proposed solutions meet the real-world needs of home security and surveillance.
- Emerging Trends and Technologies: Collaboration with industry experts allows researchers to stay informed about emerging trends, technologies, and industry innovations in the field of IDS. These experts are often at the forefront of new developments and can provide insights into the latest advancements, threat vectors, and mitigation strategies. This helps researchers align their work with industry trends and ensure the relevance and effectiveness of their research.
- Networking and Future Opportunities: Collaborating with industry experts creates networking opportunities for researchers. These connections can lead to future collaborations, joint projects, and knowledge-sharing opportunities. Establishing strong relationships with industry professionals can open doors to industry-funded research, access to industry conferences, and potential avenues for technology transfer and commercialization.
To facilitate collaboration with industry experts, researchers can attend industry conferences, participate in industry forums and workshops, and engage in networking events. Building relationships with professionals through these channels can foster collaboration and knowledge exchange. Researchers can also establish partnerships with industry organizations or security providers to access resources, data, and expertise.
By collaborating with industry experts, researchers can bridge the gap between academic research and practical implementation, leading to the development of IDS solutions that are more effective, efficient, and aligned with real-world security needs.
In the next section, we will explore another strategic approach for finding new results in IDS research: experimentation with novel techniques.
Experimentation with Novel Techniques
Experimentation with novel techniques is a crucial strategy for finding new results in IDS (Intrusion Detection System) research. This approach involves designing and implementing innovative intrusion detection algorithms, machine learning models, or data analysis techniques to address the challenges and limitations of existing approaches. Through experimentation, researchers can evaluate the effectiveness, efficiency, and practical applicability of these novel techniques in real-world scenarios.
Here are key steps and considerations for conducting experiments with novel techniques in IDS research:
- Identify Research Objectives: Clearly define your research objectives and the specific challenge or area you aim to address with your novel technique. This could include improving detection accuracy, enhancing scalability, or handling new types of attacks. Having well-defined objectives helps guide the experimental design and evaluation process.
- Design the Experiment: Define the experimental setup, including the dataset to be used, the evaluation metrics, and the performance benchmarks. It is crucial to have a diverse and representative dataset to evaluate the effectiveness and robustness of the novel technique. Define the experimental conditions, such as the network environment, attack scenarios, and data preprocessing steps, to ensure consistent and reliable experimental results.
- Implement the Novel Technique: Develop and implement the novel technique or algorithm that you aim to experiment with. This could involve designing new intrusion detection algorithms, exploring advanced machine learning models, or leveraging emerging technologies such as deep learning or anomaly detection techniques. Pay attention to the implementation details and ensure the technique is properly integrated into the IDS framework.
- Evaluate Performance: Evaluate the performance of the novel technique using appropriate evaluation metrics and benchmarks. Common evaluation metrics include detection accuracy, false positive rate, false negative rate, precision, recall, and F1-score. Compare the performance of the novel technique against existing approaches to assess its effectiveness and superiority.
- Analyze Results: Analyze the results obtained from the experiment, including the performance metrics and the behavior of the novel technique under different scenarios. Identify strengths, weaknesses, and areas for improvement. Compare your findings with existing literature and research to validate the novelty and contribution of your approach.
- Refine and Optimize: Use the insights gained from the experimental analysis to refine and optimize the novel technique. Consider feedback from the evaluation process and incorporate enhancements or modifications to address any identified limitations. Iterative refinement is crucial in improving the effectiveness and practicality of the novel approach.
- Validate in Real-World Scenarios: Once the novel technique has been refined, validate its performance and applicability in real-world scenarios. Collaborate with industry experts to obtain feedback and gather insights on the practical viability of the technique in deployed IDS systems. Real-world validation helps ensure the effectiveness and relevance of the novel technique in practical settings.
Experimentation with novel techniques provides researchers with the opportunity to explore uncharted territories within IDS research. It enables them to push the boundaries of detection performance, accuracy, scalability, and adaptability to evolving threat landscapes. Moreover, by rigorously evaluating the proposed technique, researchers can contribute new results that advance the field and provide valuable insights for the development of more robust and efficient IDS systems.
In the next section, we will explore another strategic approach for finding new results in IDS research: analysis of real-world intrusion data.
Analysis of Real-World Intrusion Data
The analysis of real-world intrusion data is a powerful strategy for finding new results in IDS (Intrusion Detection System) research. By examining actual network traffic, system logs, and attack traces, researchers can gain insights into real intrusions, understand the characteristics and patterns of malicious activities, and develop more effective detection and prevention mechanisms. The analysis of real-world intrusion data provides a practical perspective and helps researchers bridge the gap between theoretical concepts and the realities of cybersecurity threats.
Here’s a step-by-step guide to conducting the analysis of real-world intrusion data in IDS research:
- Data Collection: Collect real-world intrusion data from various sources, such as network traffic logs, system logs, and captured attack traces. Ensure the data is representative of different attack scenarios, network topologies, and system configurations. Collaborate with organizations, security agencies, or research consortia to access anonymized datasets.
- Data Preprocessing: Preprocess the collected intrusion data to ensure its quality and compatibility with your analysis techniques. This may involve data cleaning, removal of irrelevant or redundant information, and normalization of data formats. The quality of the preprocessing step directly impacts the accuracy and reliability of your analysis results.
- Exploratory Data Analysis: Perform exploratory analysis to understand the characteristics and patterns within the intrusion data. Use techniques such as visualization, statistical analysis, and data mining to uncover hidden relationships and identify potential indicators of compromise. This analysis provides insights into the nature of intrusions and helps researchers identify novel detection approaches.
- Feature Engineering: Extract meaningful features from the intrusion data that can be used to differentiate between normal and malicious activities. Feature engineering involves selecting, transforming, and creating new features that capture the essential characteristics of intrusions. This step is crucial for building accurate and effective intrusion detection models.
- Model Development: Develop intrusion detection models based on the analyzed real-world data. This could involve employing machine learning algorithms, statistical techniques, or rule-based systems. Train the models on labeled data, consisting of known intrusions and benign activities, and validate their performance using appropriate evaluation metrics.
- Evaluation and Validation: Evaluate and validate the performance of the developed intrusion detection models using various metrics, such as detection rate, false positive rate, and accuracy. Compare the results with existing detection mechanisms to assess the novelty and effectiveness of the developed models. Validation in real-world scenarios, with the collaboration of industry experts, is crucial to ensure practical viability and applicability.
- Identification of New Results: Analyze the results of the intrusion data analysis to identify new findings, patterns, or characteristics of intrusions that were previously unknown or overlooked. These new results can drive innovation and contribute to the development of more robust and accurate intrusion detection systems.
The analysis of real-world intrusion data brings a practical perspective to IDS research and helps researchers develop more effective and adaptive intrusion detection systems. By leveraging actual attack data, researchers gain valuable insights into the behavior and tactics of attackers, enabling them to stay ahead of emerging threats and contribute to the development of improved security measures.
In the next section, we will explore another strategic approach for finding new results in IDS research: the exploration of emerging technologies.
Exploration of Emerging Technologies
Exploration of emerging technologies is a vital strategy for finding new results in IDS (Intrusion Detection System) research. As the field of cybersecurity continues to evolve, researchers must stay abreast of emerging technologies and methodologies to develop more efficient and effective intrusion detection systems. By exploring emerging technologies, researchers can leverage advancements in areas such as artificial intelligence, machine learning, behavioral analysis, and threat intelligence to enhance the detection and prevention of intrusions.
Here’s how researchers can approach the exploration of emerging technologies in IDS research:
- Identify Relevant Emerging Technologies: Stay updated on the latest advancements in the field of cybersecurity and identify emerging technologies that have the potential to improve intrusion detection. This could include technologies such as deep learning, reinforcement learning, adversarial machine learning, graph analysis, or threat intelligence platforms. Research conferences, industry publications, and collaborative networks are valuable sources of information on emerging technologies.
- Understand the Technology: Dive deep into understanding the principles, algorithms, and applications of the identified emerging technology. Familiarize yourself with the underlying concepts, research papers, and case studies related to the technology. Gain a clear understanding of its strengths, limitations, and its potential application in IDS research and intrusion detection systems.
- Experimental Implementation: Experiment with the emerging technology by implementing it in IDS research. Design and develop intrusion detection models or algorithms that leverage the capabilities of the emerging technology. This could involve adapting existing models to incorporate the new technology or designing novel approaches that directly utilize the emerging technology to enhance detection accuracy or efficiency.
- Evaluation and Benchmarking: Evaluate and benchmark the performance of the intrusion detection models or algorithms that incorporate the emerging technology. Compare the results against existing approaches or state-of-the-art techniques to assess the effectiveness and novelty of the new technology-based intrusion detection systems. Use appropriate evaluation metrics and consider real-world scenarios for validation, if possible.
- Optimization and Refinement: Analyze the experimental results and feedback to optimize and refine the intrusion detection models or algorithms. Address any limitations or challenges encountered during the experimental implementation and evaluation phase. Iterate and fine-tune the models to improve their performance and adaptability in real-world environments.
- Integration and Practical Implementation: Explore the practical implementation of the intrusion detection models or algorithms that incorporate the emerging technology. Assess the feasibility of integrating them into existing IDS systems or security frameworks. Collaborate with industry experts to understand their requirements, constraints, and practical implications. Conduct pilot tests or real-world deployments to validate the applicability and effectiveness of the emerging technology-based IDS solutions.
Exploring emerging technologies provides researchers with a significant advantage in developing innovative and advanced intrusion detection systems. These technologies can enhance the detection accuracy, scalability, real-time analysis, and adaptability of IDS solutions. Furthermore, the exploration of emerging technologies allows researchers to contribute to the wider advancements in the field of cybersecurity and align their work with the latest trends and developments.
By keeping pace with emerging technologies and integrating them into IDS research, researchers can make valuable contributions to the ongoing evolution of intrusion detection systems and improve the security posture of homes, businesses, and organizations.
In the next section, we will summarize the strategies discussed and emphasize the importance of constantly seeking new results in IDS research.
Conclusion
Intrusion detection system (IDS) research is crucial for advancing the field of home security and surveillance. By constantly seeking new results, researchers contribute to the development of more robust, efficient, and adaptable IDS solutions that can effectively detect and prevent intrusions. In this article, we explored several strategies for finding new results in IDS research.
Conducting a comprehensive literature review helps researchers identify research gaps, emerging trends, and new directions in IDS. By collaborating with industry experts, researchers gain practical insights, validate their findings, and align their research with real-world security needs. Experimentation with novel techniques allows researchers to explore innovative approaches and assess their efficacy in practical scenarios. Analysis of real-world intrusion data provides valuable insights into the characteristics and patterns of intrusions, driving the development of more accurate and targeted detection mechanisms. Lastly, the exploration of emerging technologies enables researchers to leverage advancements and incorporate cutting-edge technologies into IDS systems.
The importance of constantly seeking new results in IDS research cannot be overstated. The ever-evolving threat landscape requires researchers to remain proactive and innovative in their approach. By addressing the limitations of existing IDS research, researchers contribute to improving detection accuracy, scalability, adaptability to emerging technologies, and response strategies. Additionally, seeking new results supports knowledge exchange, collaboration, and the continuous development of the security community as a whole.
In conclusion, through a combination of literature reviews, collaboration with industry experts, experimentation with novel techniques, analysis of real-world intrusion data, and exploration of emerging technologies, researchers can make significant contributions to the advancement of IDS research. By embracing these strategies and constantly seeking new results, researchers can help create a safer digital environment, protect homes and businesses from cyber threats, and ensure the continuity of the field’s evolution.
Frequently Asked Questions about How To Find New Results For Intrusion Detection System Research
Was this page helpful?
At Storables.com, we guarantee accurate and reliable information. Our content, validated by Expert Board Contributors, is crafted following stringent Editorial Policies. We're committed to providing you with well-researched, expert-backed insights for all your informational needs.