Home>Home Security and Surveillance>Why Is Anomaly Detection Vital In Each Instance Of Intrusion Detection Systems
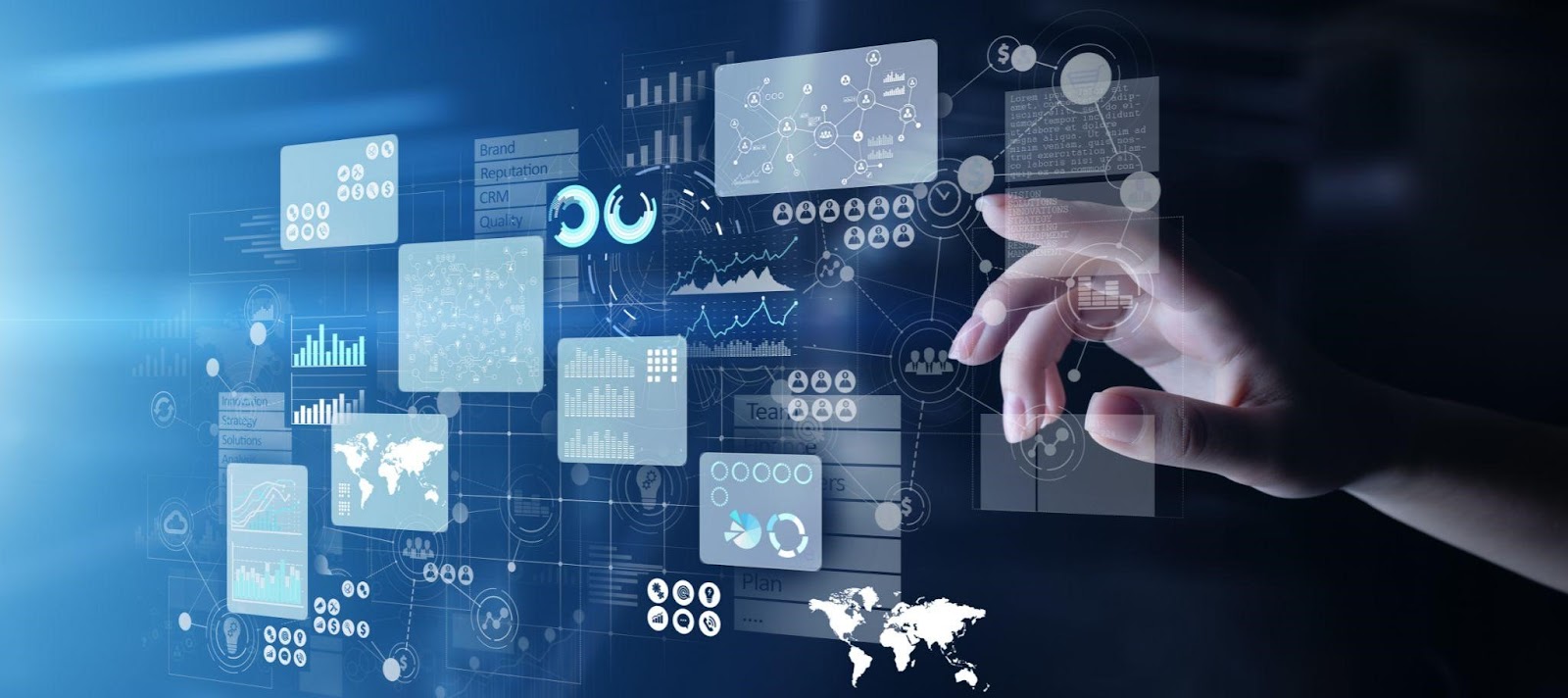
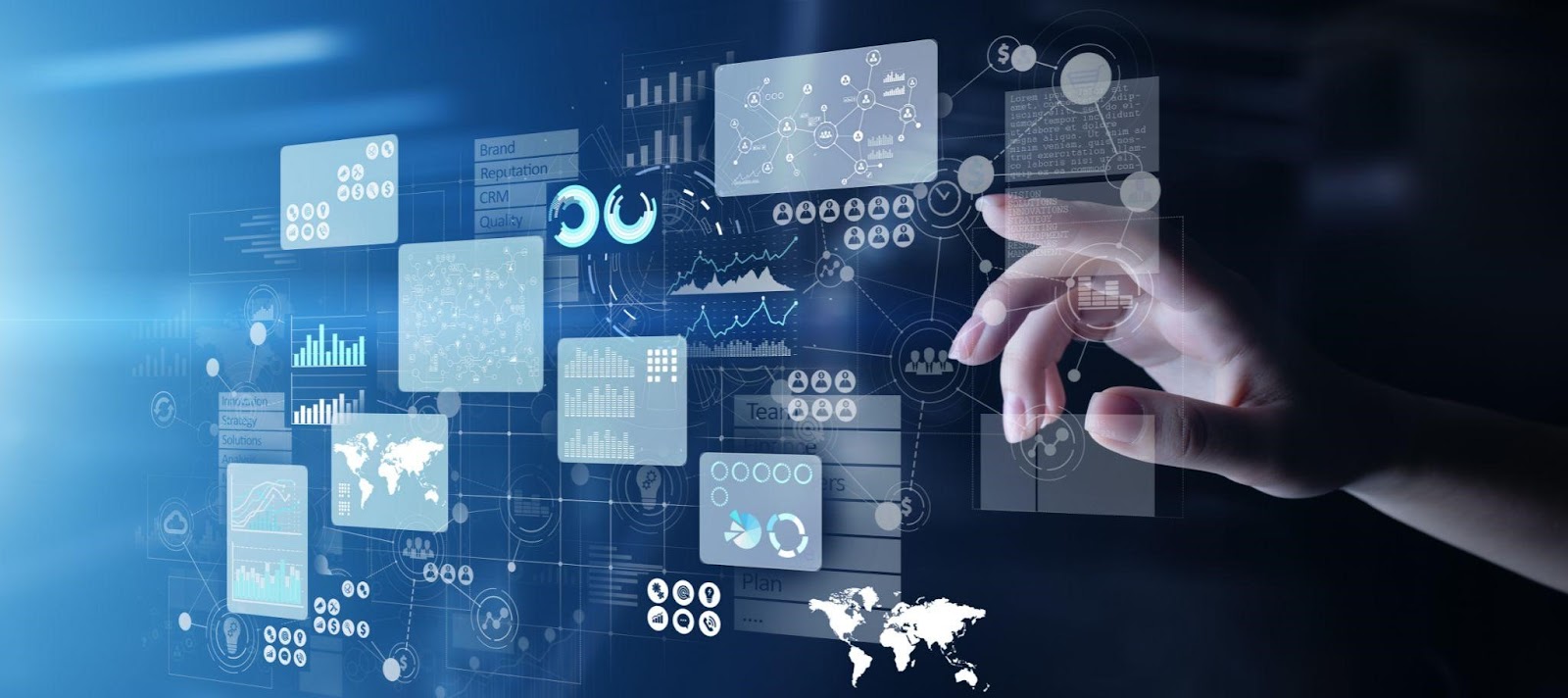
Home Security and Surveillance
Why Is Anomaly Detection Vital In Each Instance Of Intrusion Detection Systems
Modified: March 6, 2024
Discover why anomaly detection is crucial for every home security and surveillance system! Learn about the importance of intrusion detection systems and how they protect your property.
(Many of the links in this article redirect to a specific reviewed product. Your purchase of these products through affiliate links helps to generate commission for Storables.com, at no extra cost. Learn more)
Introduction
Welcome to the world of home security and surveillance! With the ever-increasing concern for personal safety and the protection of property, it’s imperative to invest in reliable security measures. One of the most effective ways to safeguard your home is by implementing a robust intrusion detection system (IDS). An IDS is a pivotal component of any comprehensive security system, designed to detect and respond to potential security breaches.
While traditional IDS primarily rely on rule-based detection methods, the emergence of anomaly detection has revolutionized the field of intrusion detection. Anomaly detection in IDS involves the identification of abnormal activities or behavior that deviate from the expected patterns. This proactive approach helps in detecting previously unknown threats and provides a higher level of security.
Throughout this article, we will explore the concept of anomaly detection in IDS and its significance in ensuring robust security for your home. We will delve into the various challenges faced in implementing anomaly detection, as well as the benefits it offers. Additionally, we will discuss some common techniques used in anomaly detection and the metrics used to evaluate their effectiveness.
By the end, you will have a deep understanding of the importance of anomaly detection in intrusion detection systems and the impact it can have on your home security.
So, let’s dive in and explore the world of anomaly detection in IDS!
Key Takeaways:
- Anomaly detection in home security systems helps identify new threats and abnormal behaviors, providing proactive protection beyond traditional rule-based methods.
- Implementing anomaly detection presents challenges, but the benefits include improved threat detection, proactive incident response, and comprehensive security coverage for homes.
Anomaly Detection: Definition and Importance
Anomaly detection is a technique used to identify patterns or activities that deviate significantly from the expected behavior within a given system. In the context of intrusion detection systems (IDS), anomaly detection plays a vital role in identifying potential security threats that may go undetected by traditional rule-based systems.
The importance of anomaly detection lies in its ability to detect unknown or emerging threats that do not conform to pre-defined rules. While rule-based systems are effective at detecting known attack patterns, they often struggle with detecting new or sophisticated attacks that may not fit into predefined rules. Anomaly detection provides a proactive defense mechanism by continuously monitoring network traffic, user behavior, or system activities to identify any unusual or abnormal patterns.
With the rapid evolution of cyber threats and the increasing sophistication of attackers, relying solely on rule-based systems is no longer sufficient. Anomaly detection fills this gap by identifying deviations from normal behavior, whether they are caused by malicious activities or system faults.
Anomaly detection is particularly crucial in the realm of home security and surveillance. Homes are vulnerable to various security breaches, ranging from break-ins and thefts to unauthorized access or intrusion attempts. By implementing anomaly detection in your home security system, you can enhance its effectiveness and your peace of mind.
Imagine a scenario where you have set specific rules for your security system, such as the time of day when the doors can be unlocked or the number of failed authentication attempts allowed. While such rules can help prevent known threats, anomaly detection adds an extra layer of security. It can detect anomalies like someone repeatedly trying to gain unauthorized access to your home or unusual behaviors outside regular hours, alerting you to potential security issues.
Furthermore, anomaly detection can also help identify system faults or malfunctions that may disrupt the normal functioning of your security system. By constantly monitoring the system’s behavior, anomaly detection can detect anomalies caused by hardware or software failures, enabling proactive measures to be taken before the security system becomes ineffective.
In summary, anomaly detection is a powerful technique that can significantly enhance the effectiveness of intrusion detection systems, particularly in the context of home security. By detecting unusual patterns, it can identify emerging threats, protect against sophisticated attacks, and help maintain the reliability of your security system.
Intrusion Detection Systems: Overview and Purpose
Intrusion Detection Systems (IDS) are critical components of a robust home security and surveillance setup. They are designed to monitor and analyze network traffic, system logs, and user activities to detect and respond to potential security breaches. IDS provide real-time monitoring, alerting, and analysis capabilities, enabling homeowners to take immediate action in response to security threats.
The primary purpose of an IDS is to identify and respond to unauthorized access attempts, malicious activities, or anomalies within a computer network or system. By monitoring network traffic and system logs, IDS can detect intrusion attempts, policy violations, malware infections, and other security breaches.
There are two main types of IDS: network-based IDS (NIDS) and host-based IDS (HIDS).
- Network-based IDS (NIDS): NIDS are deployed at strategic points within a network, such as routers or firewalls, to monitor and analyze network traffic. They examine network packets and look for suspicious or malicious activities that deviate from expected patterns. NIDS are particularly effective in detecting network-based attacks, such as port scanning, denial-of-service (DoS) attacks, or network intrusion attempts.
- Host-based IDS (HIDS): HIDS are installed on individual hosts or servers to monitor activity on those specific systems. They examine system logs, file integrity, and user activities to detect any potential signs of intrusion or malicious activities. HIDS are highly valuable for identifying insider threats, unauthorized access attempts, or abnormal system activities.
Both NIDS and HIDS play complementary roles in providing comprehensive protection against security threats. While NIDS can detect attacks at the network level, HIDS focus on detecting threats specific to individual hosts or servers.
The overarching goal of IDS is to provide early detection of security threats and facilitate a timely response. This can include generating alerts to system administrators, initiating automated countermeasures, or triggering incident response protocols. IDS not only help protect your home and its occupants but also safeguard sensitive data, prevent financial losses, and maintain the integrity of your security systems.
It’s important to understand that IDS are not foolproof and cannot prevent all security breaches. They serve as a crucial layer of defense, working alongside other security measures such as firewalls, encryption, access control, and physical security measures. By combining multiple security layers and implementing anomaly detection, IDS can significantly enhance the overall security posture of your home.
Now that we have a clear understanding of IDS and their purpose, let’s explore why anomaly detection is vital in each instance of intrusion detection systems.
The Need for Anomaly Detection in IDS
The ever-evolving landscape of cyber threats and the increasing sophistication of attackers necessitate the need for anomaly detection in intrusion detection systems (IDS). While traditional rule-based IDS are effective at detecting known attack patterns, they often struggle with identifying new or unknown threats. Anomaly detection fills this gap by providing a proactive defense mechanism that can identify novel or unusual behaviors that deviate from established patterns.
One of the primary reasons for incorporating anomaly detection in IDS is to detect zero-day attacks. Zero-day attacks are vulnerabilities or exploits that are unknown to the security community and, therefore, do not have corresponding rules in traditional IDS. Attackers constantly develop new attack methods to bypass rule-based defenses, making it imperative for IDS to adapt and detect these evolving threats. Anomaly detection can identify abnormal network traffic, unusual system behaviors, or unexpected user activities that may indicate a zero-day attack, allowing homeowners to respond swiftly and effectively.
Another crucial aspect in the need for anomaly detection is detecting insider threats. Insider threats refer to security breaches caused by individuals who have authorized access to the system, such as employees, contractors, or service providers. Unlike external attacks, insider threats can be much harder to detect as they often mimic normal user behavior. Anomaly detection can analyze user behavior patterns over time and identify any deviations that might indicate malicious intent or unauthorized activities. By including anomaly detection in IDS, homeowners can proactively identify and mitigate the risks associated with insider threats.
Furthermore, anomaly detection also helps in identifying system faults or malfunctions that may compromise the security of the home. Hardware failures, software bugs, or misconfigurations can result in abnormal system behaviors that may be exploited by attackers or cause the security system to malfunction. By continuously monitoring system activities, anomaly detection can detect these anomalies and alert homeowners to potential vulnerabilities or system health issues. This allows for timely maintenance and remediation before the security system becomes compromised.
Overall, the need for anomaly detection in IDS stems from the desire to enhance the detection capabilities beyond known attack patterns and address the challenges posed by evolving threats. By incorporating anomaly detection, IDS can provide a proactive defense against zero-day attacks, identify insider threats, and detect system faults or malfunctions, ensuring that your home remains secure in the face of emerging security risks.
Now that we understand the need for anomaly detection, let’s explore the challenges involved in implementing anomaly detection in IDS.
Challenges in Implementing Anomaly Detection in IDS
Implementing anomaly detection in intrusion detection systems (IDS) presents several challenges that need to be addressed for effective and reliable security. While anomaly detection offers a proactive approach to identifying unknown threats, there are certain obstacles that must be overcome to ensure its successful integration into IDS.
One of the primary challenges in implementing anomaly detection is the difficulty of defining “normal” behavior. Anomaly detection algorithms rely on learning patterns and behaviors that are considered normal in a given system. However, defining what is normal can be subjective and complex, as it depends on various factors such as user behavior, network traffic patterns, and system configurations. Identifying and establishing a baseline for normal behavior requires careful analysis and domain expertise to account for legitimate variations and avoid false positives.
Another challenge is the dynamic nature of systems and the need for continuous adaptation. Systems and network environments evolve over time, and what may be considered normal behavior today may change tomorrow. Anomaly detection algorithms need to adapt and learn from new data to adjust their understanding of “normal” behavior. This requires continuous monitoring, analysis, and training of the anomaly detection models to keep up with the evolving landscape of security threats and system changes.
Furthermore, anomaly detection can generate a high number of false positives if not properly tuned. False positives occur when normal behaviors are incorrectly flagged as anomalies, generating unnecessary alerts and potentially overwhelming security teams. Balancing the sensitivity of the anomaly detection algorithms is crucial to reduce false positives while ensuring that true anomalies are accurately identified. This challenge necessitates the use of proper tuning techniques and algorithms that can accurately differentiate between legitimate deviations and potential security threats.
Scalability and performance are additional challenges in implementing anomaly detection in IDS. As network traffic and system data volumes increase, anomaly detection algorithms need to process and analyze large amounts of data quickly and efficiently. This requires robust algorithms, hardware resources, and intelligent data processing techniques to handle the scalability demands of real-time security monitoring. Ensuring optimal performance without compromising the accuracy of anomaly detection is a critical aspect of successful integration into IDS.
Lastly, the interpretability of anomaly detection results can be a challenge. Anomaly detection algorithms often provide outputs in the form of anomaly scores or alerts, which must be interpreted and acted upon by security teams or homeowners. Understanding the context and severity of anomalies can be complex, and the ability to differentiate between benign anomalies and actual security threats requires expertise and experience.
Overcoming these challenges requires a combination of domain knowledge, robust algorithms, continuous monitoring, fine-tuning, and adaptation. Addressing these obstacles is crucial in harnessing the full potential of anomaly detection and ensuring its successful implementation in IDS.
Now that we have explored the challenges, let’s delve into the benefits of incorporating anomaly detection in intrusion detection systems.
Tip: Anomaly detection is crucial in intrusion detection systems because it helps identify unusual behavior that may indicate a security threat, allowing for timely intervention to prevent potential attacks.
Read more: Where To Place Intrusion Detection Systems
Benefits of Anomaly Detection in IDS
Incorporating anomaly detection in intrusion detection systems (IDS) offers numerous benefits that contribute to enhanced security and protection for your home. By identifying abnormal behaviors and deviations from established patterns, anomaly detection provides a proactive defense mechanism that complements traditional rule-based detection methods. Let’s explore some of the key benefits of anomaly detection in IDS:
1. Detection of Unknown or Emerging Threats: Anomaly detection is particularly effective in identifying previously unknown or emerging threats that may not be detected by rule-based systems. By analyzing and learning from system behavior, anomaly detection algorithms can identify abnormal activities that deviate from expected patterns. This capability enables homeowners to detect and respond to newly evolving attack techniques and zero-day vulnerabilities.
2. Early Detection of Insider Threats: Insider threats pose a significant risk to home security, as they can be harder to detect due to authorized access and mimicry of normal user behavior. Anomaly detection can analyze user actions, system logs, and behavioral patterns to identify any deviations or suspicious activities that may indicate insider threats. This early detection allows for prompt action to mitigate risks and prevent potential damage.
3. Improved Detection Efficiency: Anomaly detection enhances the detection efficiency of IDS by reducing false negatives. Traditional rule-based systems may miss sophisticated attacks that do not fit into predefined rules. Anomaly detection algorithms can identify abnormal behavior that may not match any known attack patterns, allowing for prompt detection and response to potential security breaches.
4. Proactive Incident Response: Anomaly detection in IDS enables homeowners to take proactive measures in response to potential security incidents. Upon detecting abnormal activities, IDS can generate real-time alerts, notifying homeowners or security teams to take immediate action. This proactive approach helps in minimizing the impact of security breaches and reducing the time it takes to detect and respond to threats.
5. Enhanced System Fault and Malfunction Detection: Anomaly detection can assist in identifying system faults, malfunctions, or misconfigurations that may compromise the security of your home. By continuously monitoring system behavior, anomaly detection algorithms can detect anomalous activities caused by hardware failures, software bugs, or other system issues. This early detection allows for timely maintenance and remediation, ensuring the overall reliability and effectiveness of your security system.
6. Adaptive and Self-Learning Capability: Anomaly detection algorithms can adapt to evolving threats, system changes, and user behavior over time. By continuously learning from new data, the algorithms can adjust their understanding of normal behavior, making them more robust and capable of detecting new attack patterns. This adaptability ensures that your IDS remains effective and up-to-date in the ever-changing landscape of security threats.
7. Comprehensive Security Coverage: By combining traditional rule-based detection with anomaly detection, IDS can provide comprehensive security coverage. Anomaly detection fills the gap by detecting unknown and emerging threats, while rule-based detection focuses on known attack patterns. This layered approach ensures that your home security system remains resilient against both known and unknown threats.
By incorporating anomaly detection in IDS, homeowners can benefit from increased detection efficiency, early threat detection, proactive incident response, and comprehensive security coverage. These advantages contribute to enhanced security measures, protecting your home, personal belongings, and loved ones from potential security threats.
Now, let’s explore some common techniques used in anomaly detection for intrusion detection systems.
Common Techniques Used in Anomaly Detection for IDS
Implementing effective anomaly detection in intrusion detection systems (IDS) requires the use of various techniques and algorithms. These techniques analyze network traffic, system logs, user behaviors, and other data sources to identify abnormal activities or deviations from expected patterns. Let’s explore some of the common techniques used in anomaly detection for IDS:
1. Statistical Analysis: Statistical analysis is a fundamental technique used in anomaly detection. It involves calculating statistical measures, such as mean, standard deviation, and probability distributions, to establish normal behavior patterns. Deviations from these established statistical norms can indicate the presence of anomalies. Statistical analysis can be applied to different types of data, including network traffic flows, system resource usage, or user activity logs.
2. Machine Learning: Machine learning techniques are widely used in anomaly detection for IDS due to their ability to learn from data and adapt to changing patterns. Supervised learning algorithms can be trained on labeled datasets to detect anomalies based on predefined classes. Unsupervised learning algorithms, such as clustering or density-based methods, can detect anomalies without prior knowledge by identifying patterns that deviate from the majority. Hybrid approaches that combine supervised and unsupervised learning techniques are also commonly used.
3. Time-Series Analysis: Time-series analysis is employed when dealing with data that has a sequential or temporal aspect. In IDS, this technique is useful for analyzing network traffic patterns or system log entries over time. Time-series anomaly detection algorithms model the expected behavior of the data over time and identify deviations from the established patterns. Popular time-series algorithms include autoregressive integrated moving average (ARIMA), seasonal decomposition of time series (STL), and exponential smoothing methods.
4. Data Mining: Data mining techniques involve exploring and discovering patterns or relationships in large datasets. In anomaly detection for IDS, data mining algorithms can uncover hidden insights by analyzing large volumes of network traffic, system logs, or user behavior data. This approach can identify unusual or unexpected patterns that may indicate anomalies, even if they are not explicitly defined in predefined rules or models.
5. Behavioral Profiling: Behavioral profiling builds profiles or baselines of normal behavior for users, systems, or network entities. These profiles capture typical activities, resource usage patterns, or communication behavior. Deviations from these profiles are flagged as anomalies. Behavioral profiling can be combined with machine learning techniques to adaptively update the profiles and adjust to evolving behaviors, making it effective in detecting insider threats or unusual system activities.
6. Signature-based Detection: While anomaly detection focuses on identifying unknown or unusual patterns, signature-based detection is used to detect known attack patterns or signatures. Signature-based detection relies on pre-defined rules or signatures of known attacks. When network traffic or system logs match these signatures, it indicates the presence of a specific attack. Combining signature-based detection with anomaly detection provides a comprehensive approach to detecting both known and unknown threats.
7. Ensemble Methods: Ensemble methods combine multiple anomaly detection algorithms or models to improve detection accuracy and reliability. These techniques leverage the strengths of different algorithms and their individual detection capabilities. Ensemble methods can include voting-based approaches, stacking models, or weighted combination of results to achieve better overall performance and reduce false positives or negatives.
Each of these techniques has its own strengths and limitations, and the choice of technique depends on the specific context and requirements of the IDS. Implementing a combination of these techniques can provide a comprehensive and robust anomaly detection capability for your home security system.
Now that we have explored the different techniques, let’s discuss the evaluation and performance metrics used in anomaly detection for IDS.
Evaluation and Performance Metrics for Anomaly Detection in IDS
Evaluating the effectiveness and performance of anomaly detection techniques in intrusion detection systems (IDS) is crucial to ensure their reliability and accuracy. Various evaluation metrics are used to assess the performance of anomaly detection algorithms and measure their effectiveness in detecting and distinguishing anomalies from normal behaviors. Let’s explore some common evaluation and performance metrics used in anomaly detection for IDS:
1. True Positive (TP) and True Negative (TN): True positives represent the number of correctly identified anomalies, while true negatives represent the number of correctly identified normal behaviors. These metrics measure the accuracy of the anomaly detection algorithm in correctly classifying instances.
2. False Positive (FP) and False Negative (FN): False positives occur when normal behaviors are incorrectly classified as anomalies, while false negatives occur when actual anomalies are missed or undetected. These metrics measure the errors made by the anomaly detection algorithm and the impact of false alarms or missed detections.
3. Precision: Precision, also known as positive predictive value, measures the proportion of correctly identified anomalies out of all instances classified as anomalies. It indicates the accuracy of anomaly detection by examining the percentage of identified anomalies that are actually true anomalies.
4. Recall (Sensitivity or True Positive Rate): Recall measures the proportion of true anomalies that are correctly identified by the anomaly detection algorithm. It indicates the effectiveness of the algorithm in detecting anomalies and avoiding false negatives. A high recall rate implies a low rate of missed detections.
5. F1-Score: The F1-score combines precision and recall into a single metric that provides a balanced measure of the anomaly detection algorithm’s performance. It harmonizes the trade-off between precision and recall and is particularly useful when there is an imbalance between the number of normal instances and anomaly instances in the dataset.
6. Receiver Operating Characteristic (ROC) Curve: The ROC curve is a graphical representation of the trade-off between the true positive rate and the false positive rate at various threshold settings of the anomaly detection algorithm. It visually depicts the algorithm’s performance and provides insight into its ability to classify normal instances and anomalies accurately.
7. Area Under the Curve (AUC): The AUC is a summarized measure derived from the ROC curve. It represents the overall performance of the anomaly detection algorithm, with a higher AUC indicating better performance in distinguishing anomalies from normal instances. AUC is a widely used metric for comparing and evaluating different anomaly detection algorithms.
8. Mean Time to Detect (MTTD) and Mean Time to Respond (MTTR): MTTD measures the average time taken by the anomaly detection algorithm to detect an anomaly from the time it occurs. MTTR measures the average time taken to respond to the detected anomaly. These metrics evaluate the algorithm’s efficiency in timely detection and response to security threats.
9. False Positive Rate (FPR) and False Negative Rate (FNR): FPR measures the percentage of normal instances that are incorrectly classified as anomalies, while FNR measures the percentage of actual anomalies that are missed or undetected. These metrics provide insights into the algorithm’s performance in terms of the rate of false alarms and missed detections.
10. Detection Accuracy: Detection accuracy measures the overall accuracy of the anomaly detection algorithm in correctly classifying instances as anomalies or normal behaviors. It takes into account the cumulative impact of true positives, true negatives, false positives, and false negatives.
When evaluating anomaly detection algorithms for IDS, it is essential to consider a combination of these performance metrics to gain a comprehensive understanding of the algorithm’s effectiveness, accuracy, and reliability. These metrics help assess the algorithm’s performance in detecting anomalies while minimizing false alarms and missed detections.
Now that we have explored the evaluation and performance metrics, let’s conclude our discussion.
Conclusion
In conclusion, anomaly detection plays a vital role in enhancing the effectiveness and reliability of intrusion detection systems (IDS) for home security and surveillance. By identifying abnormal behaviors and deviations from expected patterns, anomaly detection provides a proactive defense mechanism that complements traditional rule-based detection methods. It helps in detecting unknown or emerging threats, such as zero-day attacks, and provides early detection of insider threats that mimic normal user behavior.
Implementing anomaly detection in IDS presents challenges such as defining “normal” behavior, addressing system dynamics, tuning for reduced false positives, ensuring scalability and performance, and interpreting detection results. However, overcoming these challenges is crucial to harnessing the full potential of anomaly detection and ensuring its successful integration into IDS.
The benefits of incorporating anomaly detection in IDS are significant. Anomaly detection enables the detection of emerging threats, improves detection efficiency, facilitates proactive incident response, enhances system fault and malfunction detection, adapts to evolving behaviors, and provides comprehensive security coverage for your home.
Common techniques used in anomaly detection for IDS include statistical analysis, machine learning, time-series analysis, data mining, behavioral profiling, signature-based detection, and ensemble methods. These techniques leverage the strengths of different approaches to improve detection accuracy and reliability.
Evaluating the effectiveness of anomaly detection algorithms in IDS involves various performance metrics, including true positives, true negatives, false positives, false negatives, precision, recall, F1-score, ROC curve, AUC, MTTD, MTTR, FPR, FNR, and detection accuracy. These metrics help assess the performance, accuracy, and reliability of the anomaly detection algorithms.
Incorporating anomaly detection in IDS is crucial for maintaining robust and effective home security. It ensures that your security system remains resilient against evolving threats, provides early detection of security breaches, minimizes false alarms and missed detections, and allows for prompt response and mitigation. By combining anomaly detection with other security measures and best practices, you can create a comprehensive security ecosystem that protects your home, belongings, and loved ones.
So, embrace the power of anomaly detection and enhance the security of your home with a proactive and intelligent intrusion detection system. Stay one step ahead of potential threats and enjoy the peace of mind that comes with knowing your home is well-protected.
Frequently Asked Questions about Why Is Anomaly Detection Vital In Each Instance Of Intrusion Detection Systems
Was this page helpful?
At Storables.com, we guarantee accurate and reliable information. Our content, validated by Expert Board Contributors, is crafted following stringent Editorial Policies. We're committed to providing you with well-researched, expert-backed insights for all your informational needs.